september, 21 2022
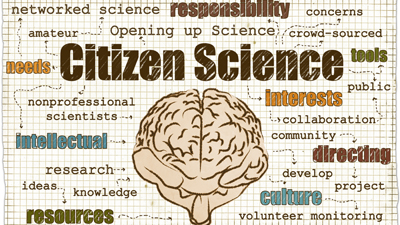
In recent times, the Citizen Science (CS) approach has been well known one of the rapid growing disciplines and practice that involves the general public in the scientific knowledge acquisition and broadening process. Research scientist have over the years emphasized that the effective use of the CS process as a method of data collection relies on techniques from many disciplines, such as science communication, informal science education, and informatics.
Recent documentation on the use of CS processes as a science has documented that citizen science can engage the public through games, peoples’ hobbies, local science and technology and does also provide sources of large and complex datasets that catalyze advances in data visualization and action research. Other organizations and researchers have also utilized the principles of the approach to ensure the successful implementation and influence of management and policy implementation under different national intervention programs.
For over a decade ago, data outcomes analyzed from citizen-science related research have been known to be characterized by biases, with a significant number originating from the extent to which people self-select to participate, their level of skill, and the spatiotemporal distribution of the data they provide. For instance, data collected by volunteers is assumed to be less accurate than data collected professionally, although the few studies that compare the precision of volunteer and professional data did not conclusively show this fact.
Alternatively, the active public involvement in scientific research has become a key source of high-quality data for scientists and policymakers. But there are concerns regarding the scientific use of citizen science data. However, it seems clear that, without proper standardized survey protocols and volunteer training, volunteer data may be highly variable in terms of precision (e.g., errors in species identification or biases in count estimates, uneven sampling effort, both in terms of temporal and spatial coverage, among others).


CS projects are prone to providing data that are spatially biased toward more densely populated, easily accessible habitats, and sampling efforts in different localities of the issues under interrogation may not reflect actual occurrences. That notwithstanding, the summative quality of data collected by volunteers, on a project-by-project basis, has generally been found as reliable as the data collected by professionals in community-based research usually cut across different subject and territories of their experiences.
Several advances have been central in the development, vetting, and dissemination of citizen-science datasets that have allowed for new areas of scientific research that would not otherwise be possible; particularly questions requiring data collected over broad spatiotemporal scales. Undeniably, a series of citizen-science programs have operated for decades across continents and countries with a series of researchers relying on the knowledge, wisdom and experiences of locals to build a long-lasting resolution to scientific hypothesis and objectives on different topics of interest.
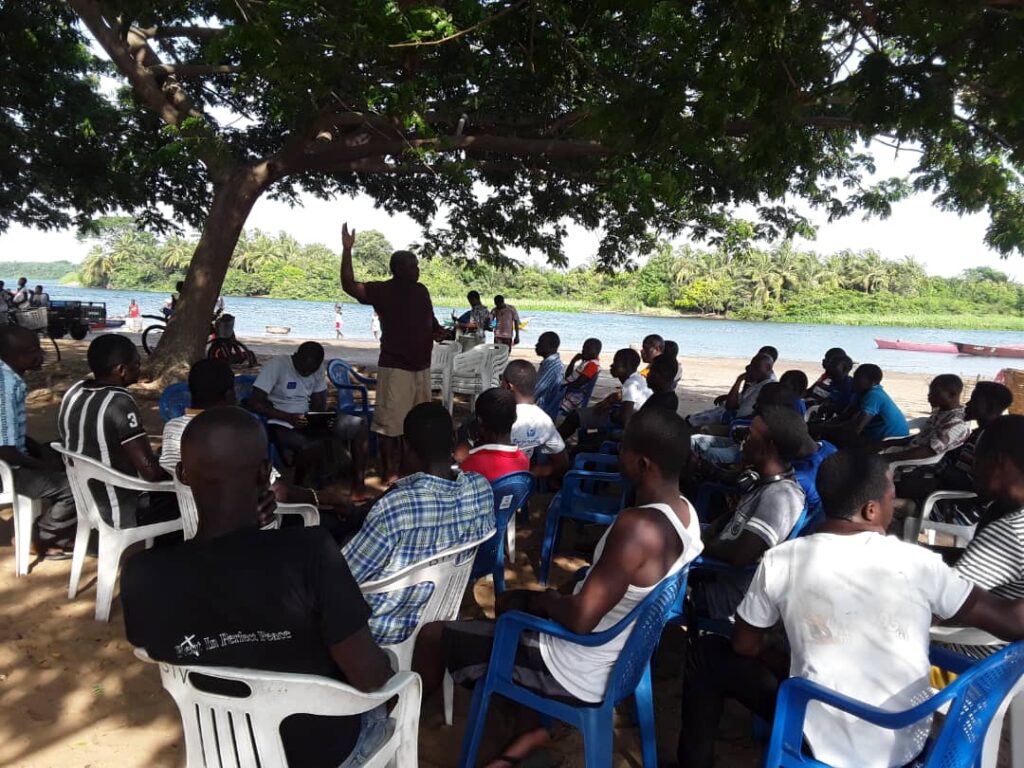
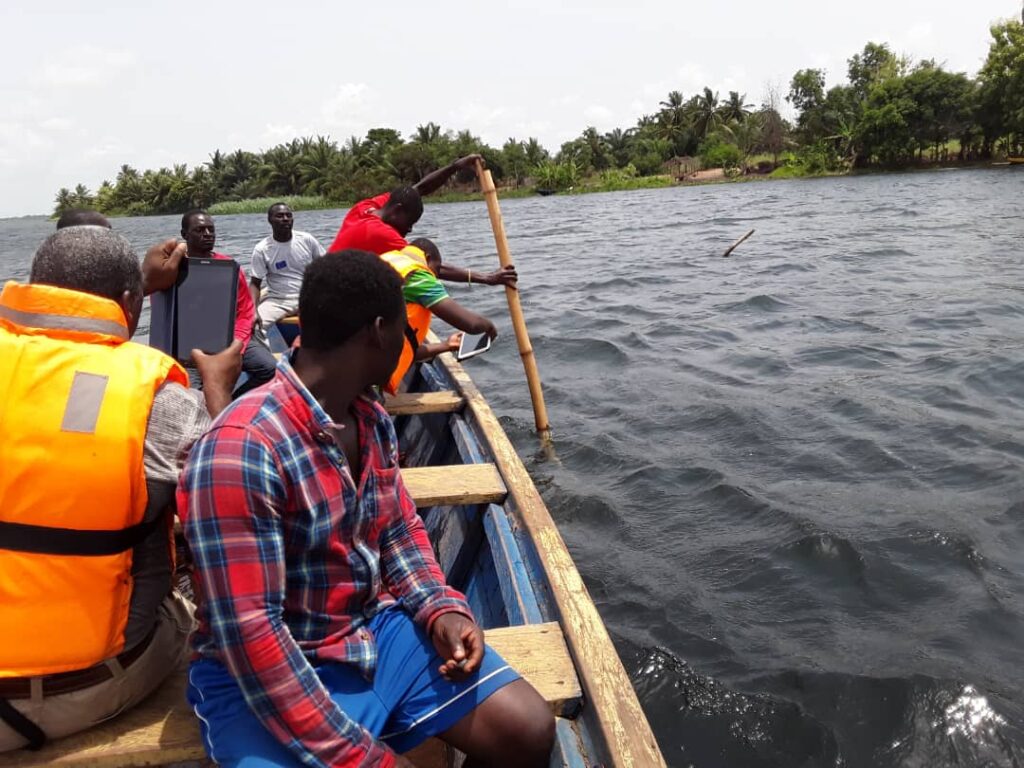
Research topics explored using CS spans from climate change and climate induced impact, environment and resource management (forest and wildlife conservation science), governance, land and agriculture management, and well as water management issues. For instance, for its application in conservation science, the involvement of the public in scientific research, has become increasingly important in conservation science as it provides an opportunity to use large numbers of observers to address issues over broad geographic areas and for long time periods.
Clearly, the benefit of such information would otherwise be impossible to collect due to time, resource constraints, and poor understanding of the local terrain. Relatedly, such data have fruitfully been used to address questions regarding patterns of species occurrence diversity patterns, bird distributions, migration, inventory and monitoring. Furthermore, despite its successes in the use of CS approaches for conservation science as an example, there is an increasing availability of large-scale environmental data (e.g., satellite imagery), which can be used to understand the relationship between human mobility and migration within Sub-Saharan Africa.

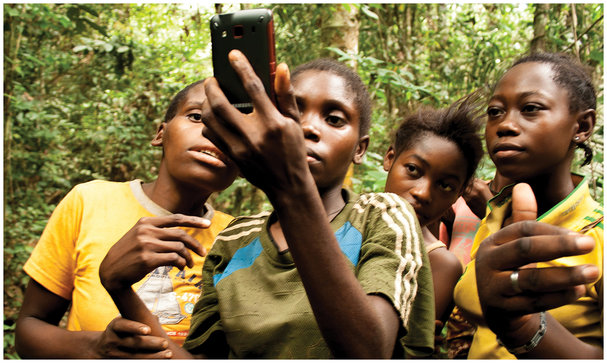
In the adoption of CS approach under the MIGRAWARE project, we demonstrate that the approach could be used to identify migration interest and intent, environmental factors and target’s location for migration intentions. While focusing on the possibility of spatial biases, it is hoped that the application of this approach will provide a different dimension that are challenging to achieve using the traditional data collection approach. In conclusion together, our goal was to evaluate the use and confidence of citizen science in advancing understanding in an important area of migration and climate change.